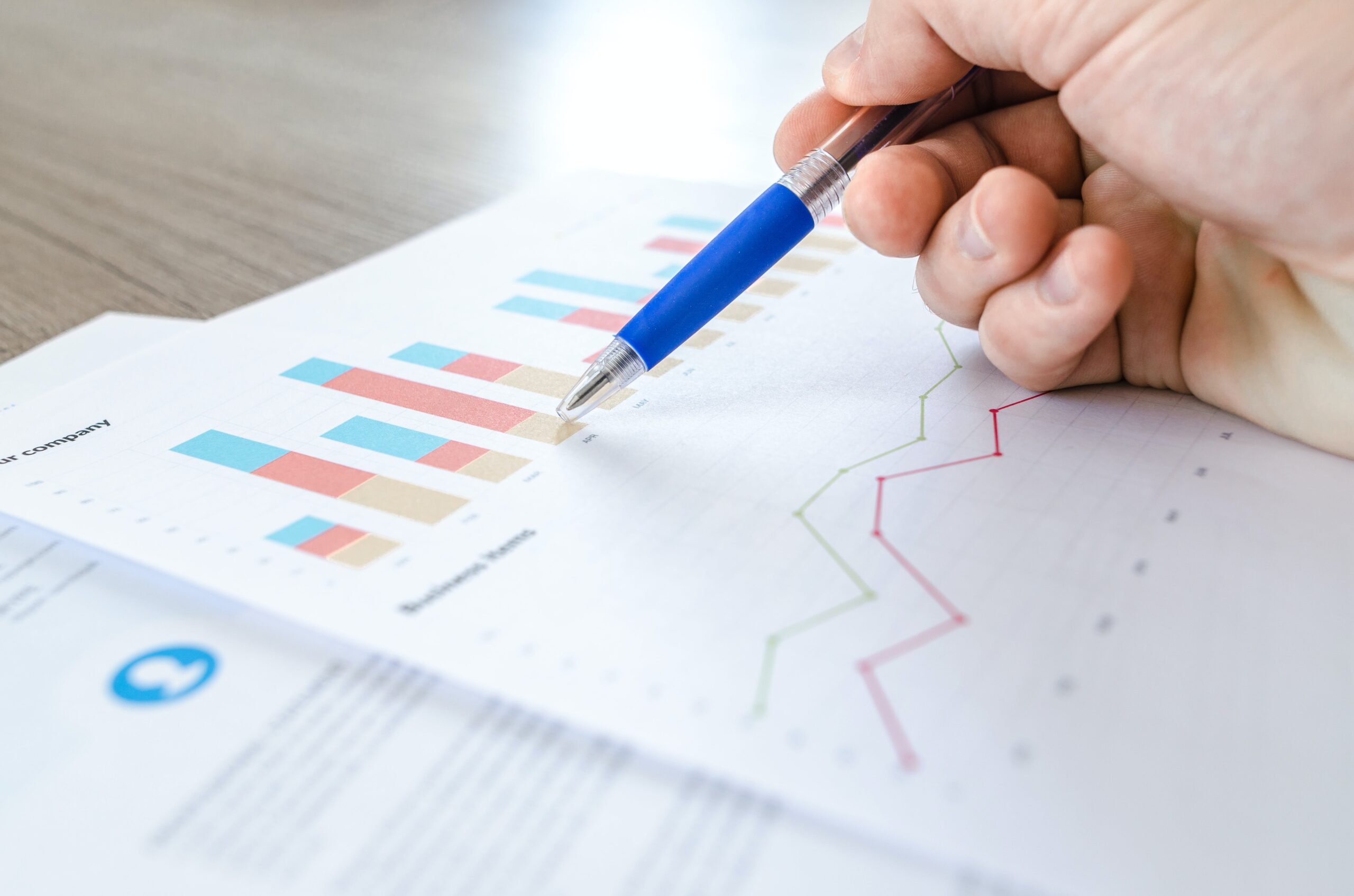
About Course
Monitoring and Evaluation (M&E) is a crucial aspect of data science projects to ensure their success and effectiveness. M&E involves systematically tracking and assessing the progress and impact of a project, allowing for informed decision-making and continuous improvement. Here’s how M&E can be applied in data science:
1. Define Clear Objectives and Key Performance Indicators (KPIs):
- Clearly define the objectives of your data science project.
- Identify measurable KPIs that align with your goals and objectives.
2. Establish Baselines:
- Establish baseline metrics before the implementation of your data science solution.
- Baselines provide a reference point for evaluating the impact of your project.
3. Data Collection and Quality Assurance:
- Implement data collection processes to gather relevant information.
- Ensure data quality through validation, cleaning, and verification procedures.
4. Real-time Monitoring:
- Implement real-time monitoring to track the performance of your data models and algorithms.
- Use visualization tools and dashboards to monitor key metrics.
5. Regular Reporting:
- Generate regular reports to communicate project progress.
- Include key insights, challenges, and recommendations for improvement.
6. Feedback Loops:
- Establish feedback loops for continuous improvement.
- Gather feedback from stakeholders, end-users, and project team members.
7. Model Evaluation:
- Regularly evaluate the performance of your machine learning models.
- Use metrics such as accuracy, precision, recall, and F1 score to assess model effectiveness.
8. Adaptability and Iteration:
- Be prepared to adapt your data science solution based on monitoring findings.
- Iterate on models, algorithms, or processes to improve performance.
9. Stakeholder Engagement:
- Engage with stakeholders to understand their needs and expectations.
- Communicate M&E findings in a way that is understandable to both technical and non-technical stakeholders.
10. Risk Management:
Student Ratings & Reviews
No Review Yet